Interactive Imitation and Contrastive Learning
Under Review
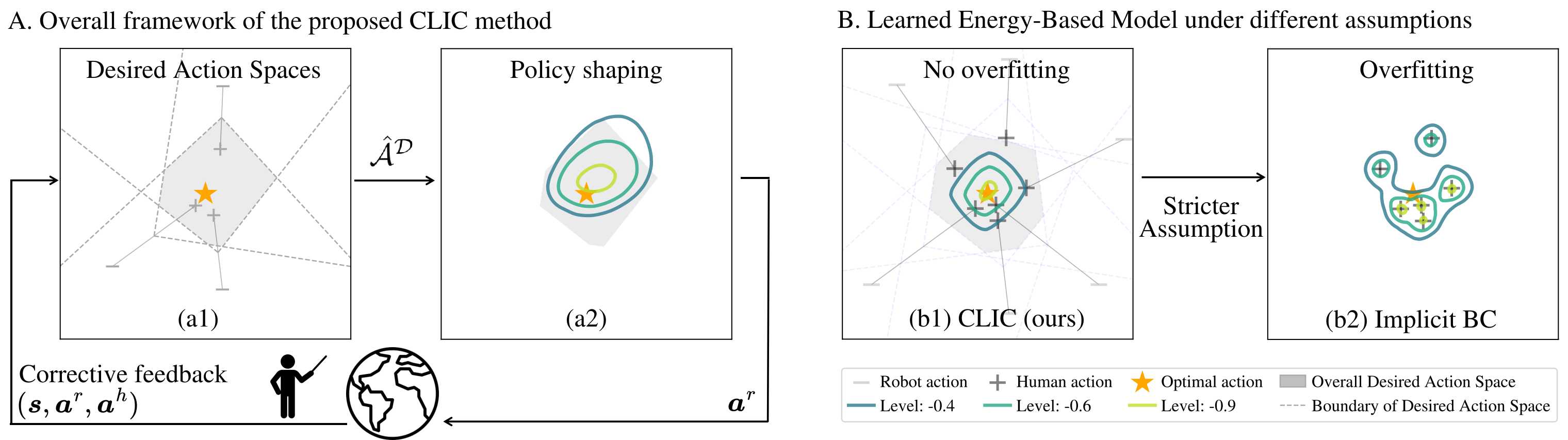
Abstract. Behavior cloning (BC) traditionally relies on demonstration data, assuming the demonstrated actions are optimal. This can lead to overfitting under noisy data, particularly when expressive models are used (e.g., the energy-based model in Implicit BC). To address this, we extend behavior cloning into an iterative process of optimal action estimation within the Interactive Imitation Learning framework. Specifically, we introduce Contrastive policy Learning from Interactive Corrections (CLIC). CLIC leverages human corrections to estimate a set of desired actions and optimizes the policy to select actions from this set. We provide theoretical guarantees for the convergence of the desired action set to optimal actions in both single and multiple optimal action cases. Extensive simulation and real-robot experiments validate CLIC's advantages over existing state-of-the-art methods, including stable training of energy-based models, robustness to feedback noise, and adaptability to diverse feedback types beyond demonstrations.
Highlights
The iterative process of CLIC involves shrinking the desired action space (gray) while updating the EBM accordingly.
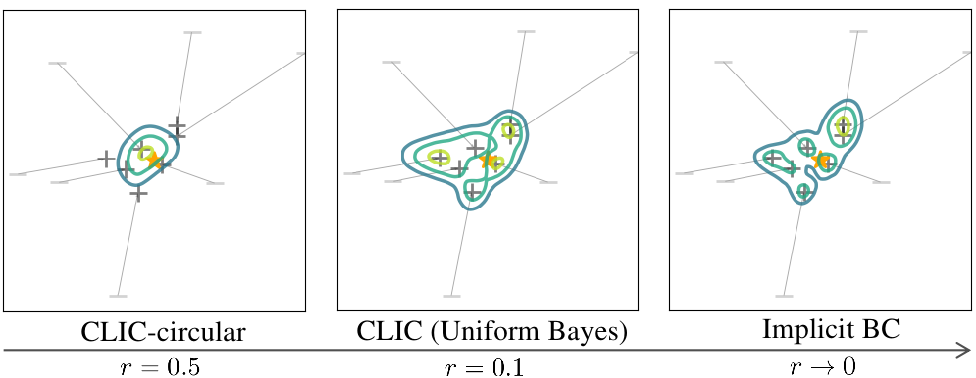
CLIC with circular desired action spaces reduces to Implicit BC when the raduis going to zero, and the policy-weighted loss is replaced by a uniform Bayes loss.
Ball catching: Quick coordination with
partial feedback to the end effector
or the robot hand.
Water pouring: Learning full pose
control to precisely pour
liquid (marbles) into a bowl.
Insert-T: Learning long-horizon multi-
modal task that inserts the T-shape
object into the U-shape object.
Simulation benchmarks
Our CLIC method outperforms prior state-of-the-art on 4 tasks, where we consider various feedback types for each task. Examples of the trajectory rollout of CLIC method (after training) using accurate demonstration are shown in the video below:
Real World Insert-T Task
The Insert-T task requires the robot to insert a T-shaped object into a U-shaped object by pushing. We categorize the task into three difficulty levels, and examples of the post-training policy rollouts for each level are shown below:
Insert-T 'Easy' category:
Insert-T 'Medium' category:
Insert-T 'Hard' category:
Team
Omited for anonymous review.
Acknowledgements
Omited for anonymous review.